No pain, still gain - Virtual Contrast could make it possible
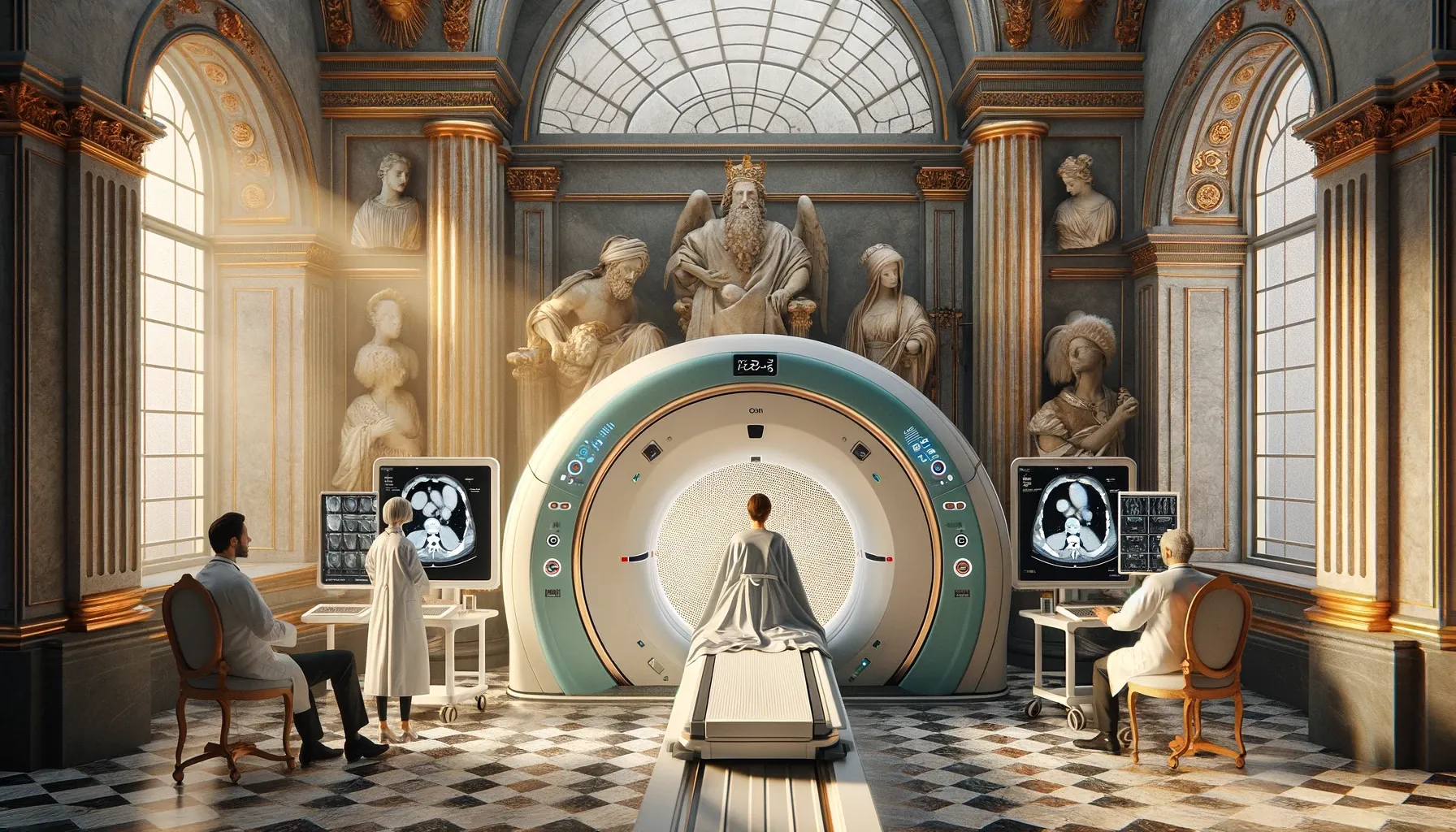
Revolutionizing Radiology: GANs Pave the Way for Safer, Virtual Contrast-Enhanced CT Scans
In a fascinating development in medical imaging technology, Jingya Liu and colleagues have introduced an innovative method that could revolutionize how contrast-enhanced CT scans are performed. Published in the notable journal 'Computational Medicine and Imaging Graphics', their research unveils a generative adversarial network (GAN) framework capable of creating virtual contrast-enhanced CT images directly from non-contrast scans.
Why This Matters
Traditionally, contrast-enhanced CT scans require the injection of contrast agents that, although effective in enhancing image quality, come with risks. Allergic reactions and nephrotoxicity pose significant health threats, especially to patients with compromised kidney function. Liu's research proposes a game-changing solution: simulate the contrast enhancement digitally, bypassing the need for physical contrast agents.
Innovative Approach
The study's brilliance lies in its dual-path framework, which meticulously synthesizes high-resolution images by capturing both the macro structures and intricate textural details of the abdomen and pelvic regions—areas particularly challenging due to their complex anatomy.
Core Innovations of the Study Include:
- Dual-Path Framework: This method processes images through two pathways to capture both global anatomical structures and local textural details, enhancing the accuracy of the virtual contrast.
- Perceptual Loss Function: Moving beyond traditional loss functions, this approach utilizes perceptual loss to better grasp high-level semantic differences between the enhanced and original images, refining the synthesis of virtual contrast.
- Self-Supervised Pretraining: To address the challenge of limited training data, the framework employs a self-supervised pretraining strategy on a large dataset, improving the model’s robustness and effectiveness.
Implications for Patient Safety and Healthcare
By eliminating the need for contrast agents, this technology promises to reduce the exposure to potential side effects associated with these chemicals, offering a safer alternative for patients needing frequent scans. Moreover, it stands to significantly cut down healthcare costs by minimizing the dependency on expensive and potentially hazardous substances.
Read and Discover More
For those intrigued by the technical depths of this research or its implications on future medical practices, the full paper details every nuance of the methodology and findings. This study is not just a technical achievement; it's a beacon for future innovations that prioritize patient safety and push the boundaries of medical imaging technology.
Explore the full research here.